题 目: Deep Learning Methods for Multiscale Mechanical Problems
报告人:Zhen Li Clemson University
时间:2024年6月27日星期四下午13:30
地点:上海大学延长校区力学所200学术报告厅
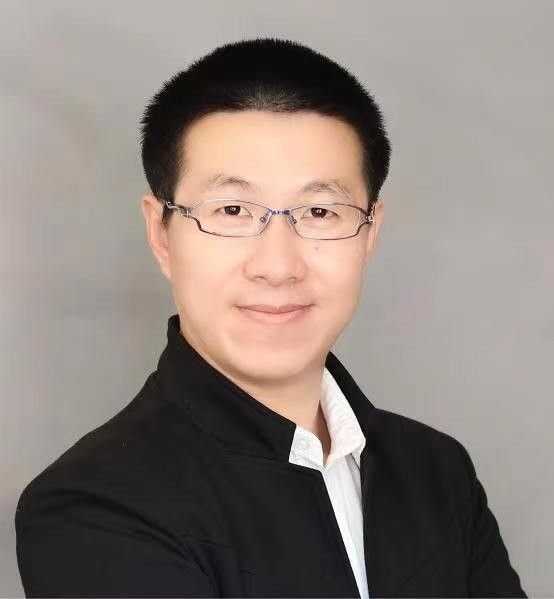
专家简介
Dr. Zhen Li is Assistant Professor of Mechanical Engineering at Clemson University. He received his B.S. in Engineering Mechanics from Wuhan University in 2005 and Ph.D. in Fluid Mechanics from Shanghai University in 2012. After a short postdoctoral experience in University of California-Merced, he joined the CRUNCH group at Brown University in 2013 as a postdoc and then was promoted to research assistant professor in 2016 and research associate professor in 2019. His research focuses on multiscale modeling for tackling challenges in multiscale/multi-physics problems, in particular on mathematical and physical foundations of scale-bridging, multiscale modeling methods and machine-learning approaches for complex fluids, biophysics and soft matter physics, which are supported by DOE, NSF, NASA and ARL projects. Dr. Li has published more than 60 journal papers, and released multiple open-source software packages, including the MUI library, the USERMESO GPU-accelerated particle simulator and the DPD-MESO module of LAMMPS.
摘要信息
Intrinsic multiscale features in various physical systems stem from hierarchical structures that span a broad spectrum of temporal and spatial scales beyond the reach of any single simulation method, which have been recognized as significant challenges in multiscale engineering problems. Recent advancements in deep learning, particularly in deep neural networks, have shown remarkable successes in different scientific research fields. In this talk, I will introduce three deep learning approaches applied to tackling multiscale challenges in mechanical engineering problems, including a multiscale neural operator network model designed for inferring bubble dynamics across scales from the nanoscale to macroscopic continuum scale, a deep neural operator model that functions as an effective surrogate for physics-based finite element models to predict the transient response of composite materials under dynamic loading conditions, and combined neural operator learning with physics-informed symbolic regression for model discovery directly from observational data.